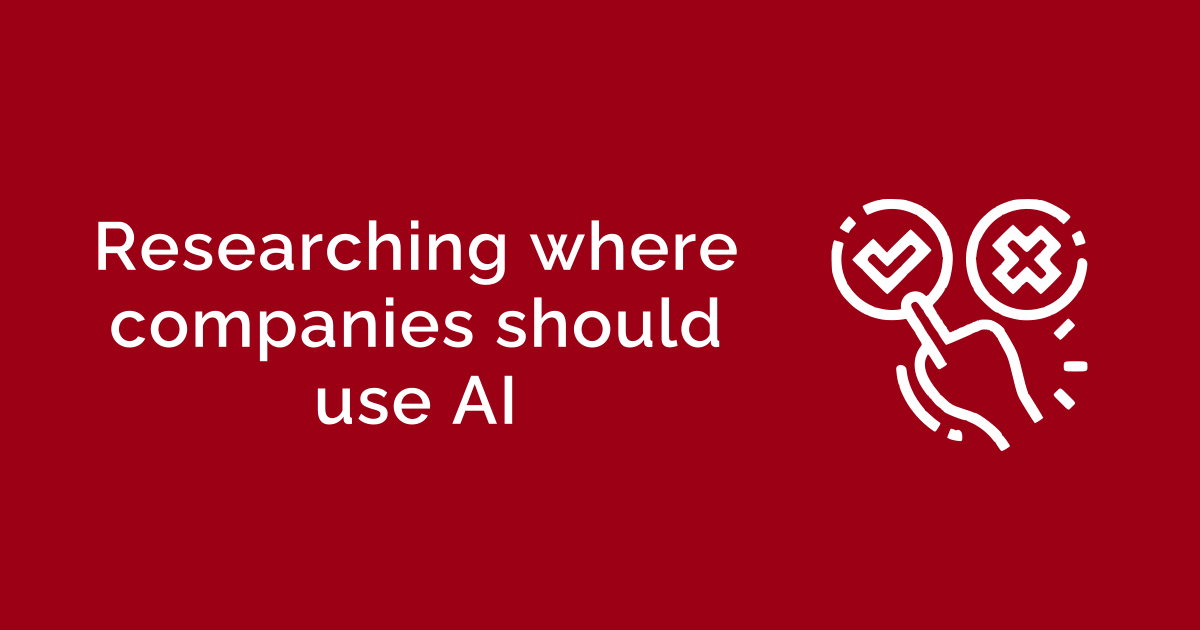
If the only tool you have is an hammer, it is tempting to treat everything as if it were a nail.
Abraham Maslow, 1966
The main objective of my masters’s thesis was to explain to MBA professors and people without a technical background, for what kind of decisions companies could make the most from Artificial Intelligence (AI) applications.
However, this hasn’t been the objective since day one. Instead, it slowly emerged while reading dozens of scientific publications on the topic. To address this unanswered question, I organized interviews with data executives (CDOs, CTOs, Directors of Analytics…) to understand where their companies are currently employing AI, why, and what they plan for the future.
I worked on the research between February and October 2023, concurrently with my internship at Accenture in Milan. To keep myself accountable for progressing a bit every day, I tracked in a spreadsheet my daily commitment, unaware that streak habits apps were a thing.
In the end, I was able to submit the work in time for the Autumn graduation. One month later, I defended it in front of my professors, family, and friends.
But let’s see how it all started.
Coming up with an interesting topic
In our faculty, most students choose their thesis topics from a list of proposals that our professors publish in March. But for Double Degree students like me, we had to propose a research topic in February, and our faculty administration would assign us an interested supervisor.
After studying Business Intelligence at ESC Clermont Business School, I became interested in the topics of predictive analytics and prescriptive analytics, the processes of analyzing data to predict future outcomes and to obtain business recommendations, respectively.
During the first meeting with my supervisor, he introduced me to the advantages of a bibliometric analysis compared to a traditional systematic literature review. While the latter is used to summarize the existing literature of a limited research field, a bibliometric analysis can identify the main research themes, their evolution, and the most influential authors in a broad research field, analyzing metadata correlations in a large list of publications.
Business decisions are not always made based on digital information: competitive dynamics, long-term objectives, strategies, and values are just a few of the factors inaccessible to information systems. Prescriptive analytics can generate possible alternatives to choose based on additional, non-digital information. However, since Scopus was listing only 900 publications related to prescriptive analytics compared to 32k of predictive analytics, I started to question where the term prescriptive analytics originated from, finding the answer in a paywalled Gartner paper12.
Together with my supervisor, we decided to take a step back and perform an exploratory analysis of the data-driven decision-making field. I would then describe how the literature evolved, and then pick a recent theme to analyze more deeply.
Understanding current research themes
The following Scopus query returned a list of 20k articles from 1982 to 2023, which I downloaded as .csv and opened with Bibliometrix:
data AND driven AND decision AND making
AND ( LIMIT-TO ( DOCTYPE , "ar" ) )
AND ( LIMIT-TO ( SUBJAREA , "BUSI" ) )
AND ( LIMIT-TO ( LANGUAGE , "English" ) )
AND ( LIMIT-TO ( SRCTYPE , "j" ) )
With Bibliometrix It was easy to see how research themes evolved based on the keywords of each paper. The few publications in the 80s were covering databases (SQL was standardized in 1987) and decision support systems, while in the following years the research diverged into separate areas: expert systems, e-services, data mining. Over the years, these separate areas merged into three highly-interconnected clusters: big data, innovation, and artificial intelligence (AI).
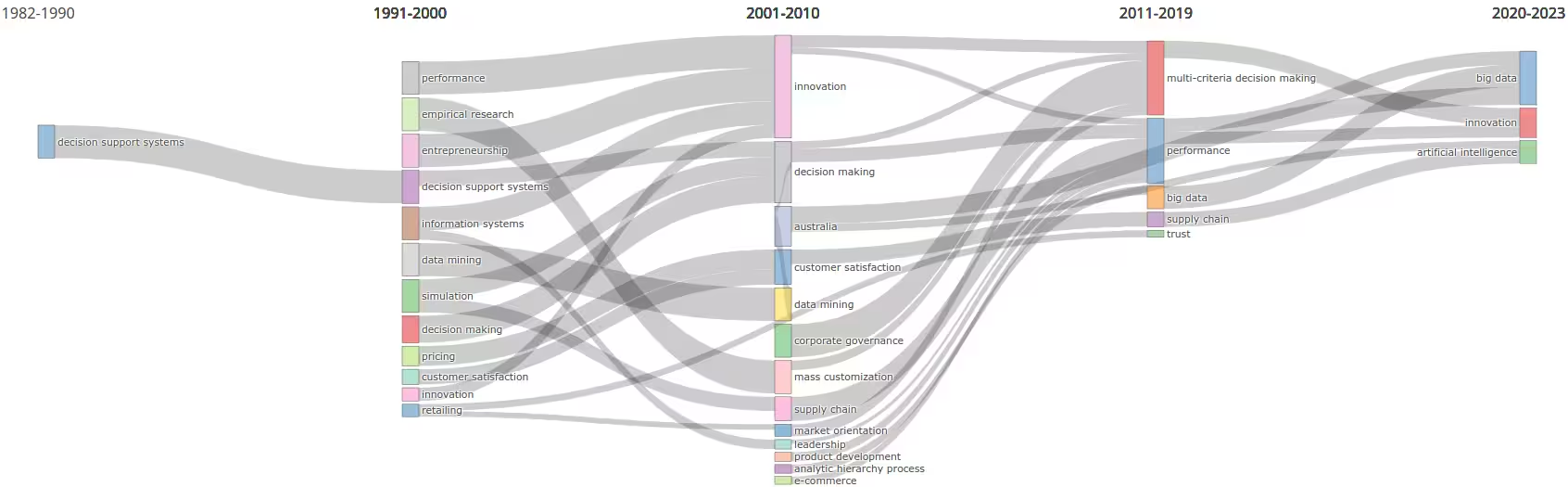
I then decided to narrow down the AI cluster, given its relevance in the current period3. Everybody was crazy about ChatGPT.
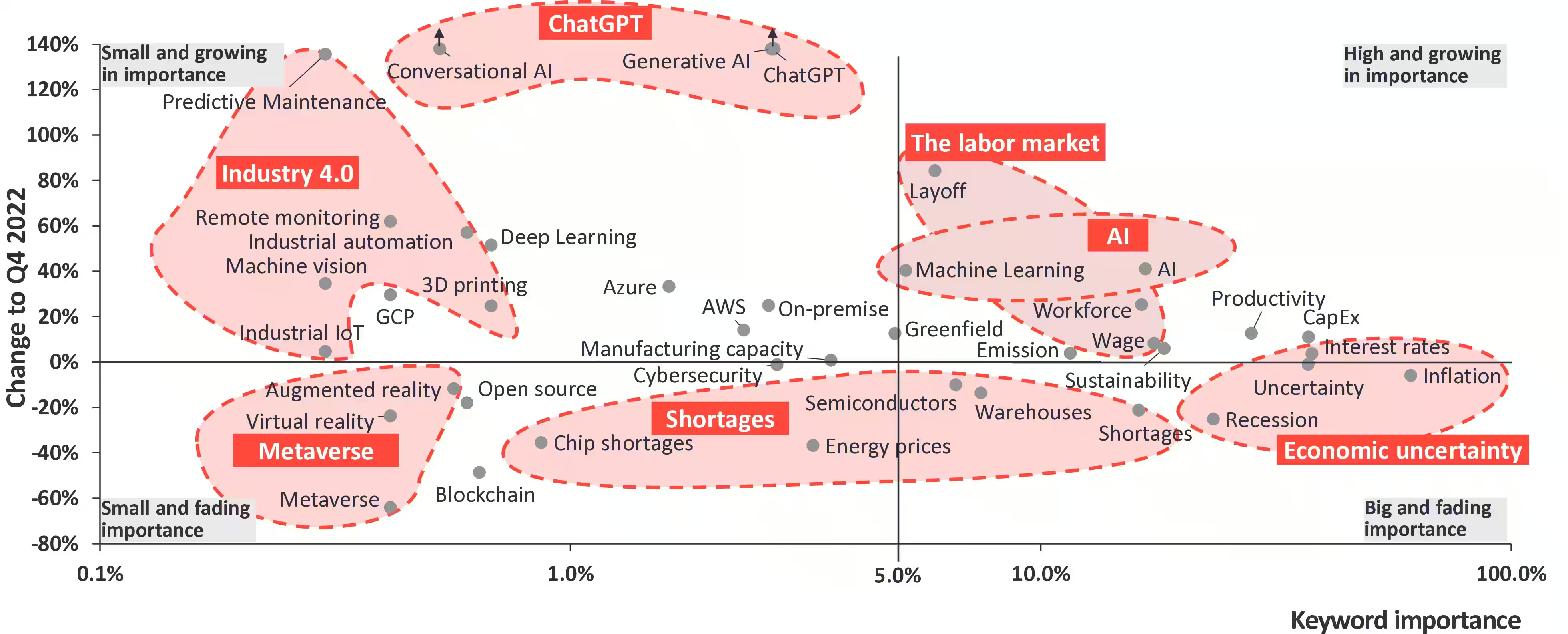
artificial AND intelligence AND decision AND making
AND PUBYEAR > 2019
AND ( LIMIT-TO ( DOCTYPE , "ar" ) )
AND ( LIMIT-TO ( SUBJAREA , "BUSI" ) )
AND ( LIMIT-TO ( LANGUAGE , "English" ) )
AND ( LIMIT-TO ( SRCTYPE , "j" ) )
This time I also selected two additional columns before exporting, to include the references cited (52k entries) and citations received (9k entries) for each article. With VOSViewer I used these attributes to derive two graphs:
- what past publications influenced the current literature on AI in decision-making, from the similarity of the references cited
- how current literature is organized, from the similarity of the citations received
The main themes of past publications are fuzzy sets (red), machine learning (ML) (green), business applications of AI (blue), behavior, bias, and aversions (yellow), uncertainty (purple) and data analytics (cyan). Past publications belonging to the fuzzy sets and uncertainty clusters tend to be cited together with similar publications, while the same is not so evident for other themes. This means that current literature on fuzzy sets is very distant from the literature on other AI themes.

The main themes of the current literature instead are explainable AI (red), multi-criteria decision-making (MCDM) (green), AI in operations (blue), robotics (yellow), AI in decision-making (purple), AI in human resources (cyan), fuzzy sets (orange), and AI in innovation (brown). Also in this case, literature in fuzzy sets and MCDM appears more technical and thematically distant from other research areas.
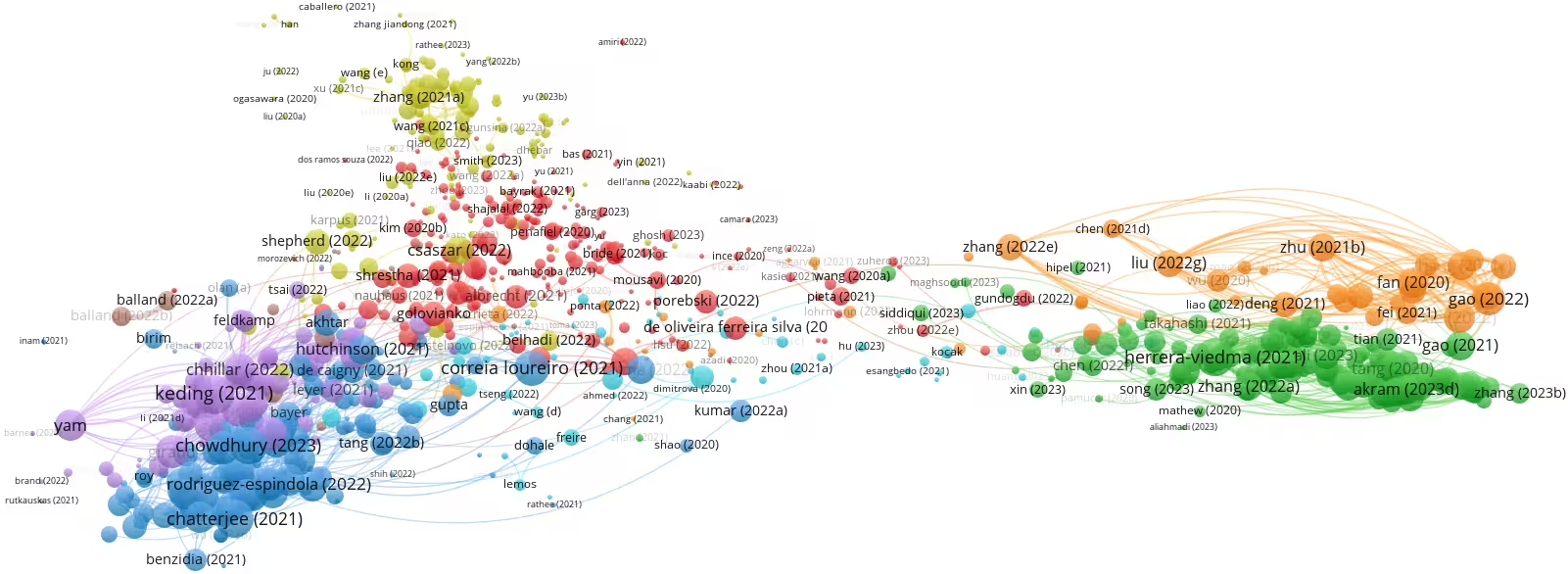
Finding a gap by playing Monopoly
By reading the most influential publications for each cluster, I explained how AI went from a rule-based approach (expert systems, e.g. Wizards) to an example-based one (machine learning, e.g. Computer Vision).
In a paper4 on the implementation of a Monopoly-playing deep Learning agent, the authors highlighted how their hybrid decision-making approach was taking advantage of predefined rules and iterative learning, resulting in a faster win rate convergence compared to pure deep-learning agents.
To achieve so, the authors:
- addressed infrequent decisions with a rule-based policy (e.g. always accept a trade that increases the number of monopolies)
- simplified complex decisions by reducing the set of possible solutions (e.g. price offers can be either 25% below, 25% up, or equal to market value)
Instead of letting the agent explore the rare state-action pair, it may be better suited if these are replaced by rule-based logic, especially if we know what actions might be good in the given state.
I had an epiphany: if not all business decisions are alike, for which ones it makes sense to use AI? When it is better to make decisions manually? By reading again papers from the literature, there was no clear answer to this question. However, I collected common decision proprieties to derive a spectrum (not a dichotomy) of business decisions, and named the extremes strategic decisions and operational decisions.
Strategic decisions | Operational decisions | |
---|---|---|
Focus | Long-term | Short-term |
Importance | High | Low |
Frequency | Low | High |
Complexity | High | Low |
Structurability | Low | High |
Dependency on data | Low | High |
Considering this framework, the research question became:
- Is AI more suited for operational rather than strategic decision-making?
Now, it was time to collect some data to argue an answer.
Where AI is being used today
Time was running short, and my supervisor agreed that having 1:1 interviews with data executives would lead to more insights than an anonymous survey. To restrict the interview sample, I opted for product companies whose core business is not AI-related. Ideally, if they adopted AI applications as part of their digital strategy, they could explain what advantages that AI adoption brought.
So I started to reach out to my network, HR departments of large companies, and cold email and LinkedIn messages. Out of 94 invitations, only 10 executives agreed to be interviewed. I asked everybody these four main questions:
- For what decisions are you employing AI applications, such as Machine Learning or Expert Systems?
- Why did you opt for AI applications, and what were the alternatives you considered?
- For what decisions are you not employing AI applications, and why not?
- Do you think that these challenges will persist in the future?
Surprisingly, no company provided an example of a strategic decision taken with AI, not even the more technologically advanced. Most of the respondents cited employee productivity, telemetry data analysis, optical pattern recognition, time-series forecasting, and embedded AI-based features in their product offerings.
As the main reasons for AI adoption, they mentioned availability of data, possibility to automate the decision, employee productivity boost, accuracy in forecasting and pattern recognition, and the capacity to improve from new examples.
The main reason why they did not adopt AI for more strategic decisions, is that AI has limited cognitive capabilities. Few of them also cited stakeholders’ or managers’ reluctance towards AI automation. Regarding AI non-adoption for other, more operational decisions, they mentioned the need for accurate results, complexity of decisions, cost-benefit trade-offs, and the maintenance costs of ML models.
As considerations for future AI employment in more strategic decisions, most of them want to limit AI to narrow or data-intensive tasks, as it cannot take into account all the needed factors to make such decisions. Regarding more operational decisions, they expressed a desire to upskill employees, spread a data-driven mentality and stay current with novel use cases.
According to the interview sample, the decisions that can benefit the most from AI employment present one or more of the following proprieties: they can be taken from data that is easily collectible or already available, they can be structured and automated, they do not require human-level cognition, they are narrow in their scope, and they happen routinely. Companies value AI technologies for their accuracy in forecasting, accuracy in pattern recognition, fast results compared to large computations, and their capacity to learn from examples.
Therefore, we can conclude that AI is more suited for operational decisions rather than strategic ones.
Acknowledging research limitations
I was satisfied with the results, but I had to acknowledge the limitations of my research before submitting it. First, qualitative interviews did not allow for a larger sample size and less biased answers. Second, by not restricting the sample to a specific industry, geography, or company size, the results were less comparable. Another criticism that could be raised is that individual executives of large companies may be unaware of other AI use cases inside the same company.
However, I was able to collect a tremendous amount of insights from a few qualitative interviews, many companies reported the same findings regardless of their size or industry, and I reached out to the most aware representatives I could interview.
Presenting the research
I finalized my slide deck at 9 AM the day of the dissertation, after an all-nighter, several remakes, and endless LaTeX runs. Picking up the hard-cover book of the thesis from the shop gave me the confidence that I did a great job. Now it was just time to rest a bit, attend a friend’s dissertation, and try to not forget what to say.
Around 2.30 PM relatives and friends joined me outside of the faculty building. Seeing all these people coming just for me, my body kicked in all the adrenaline. After the professors called my turn, I took a deep breath, grabbed my USB laser pointer and speech timer, and gave my best speech ever.
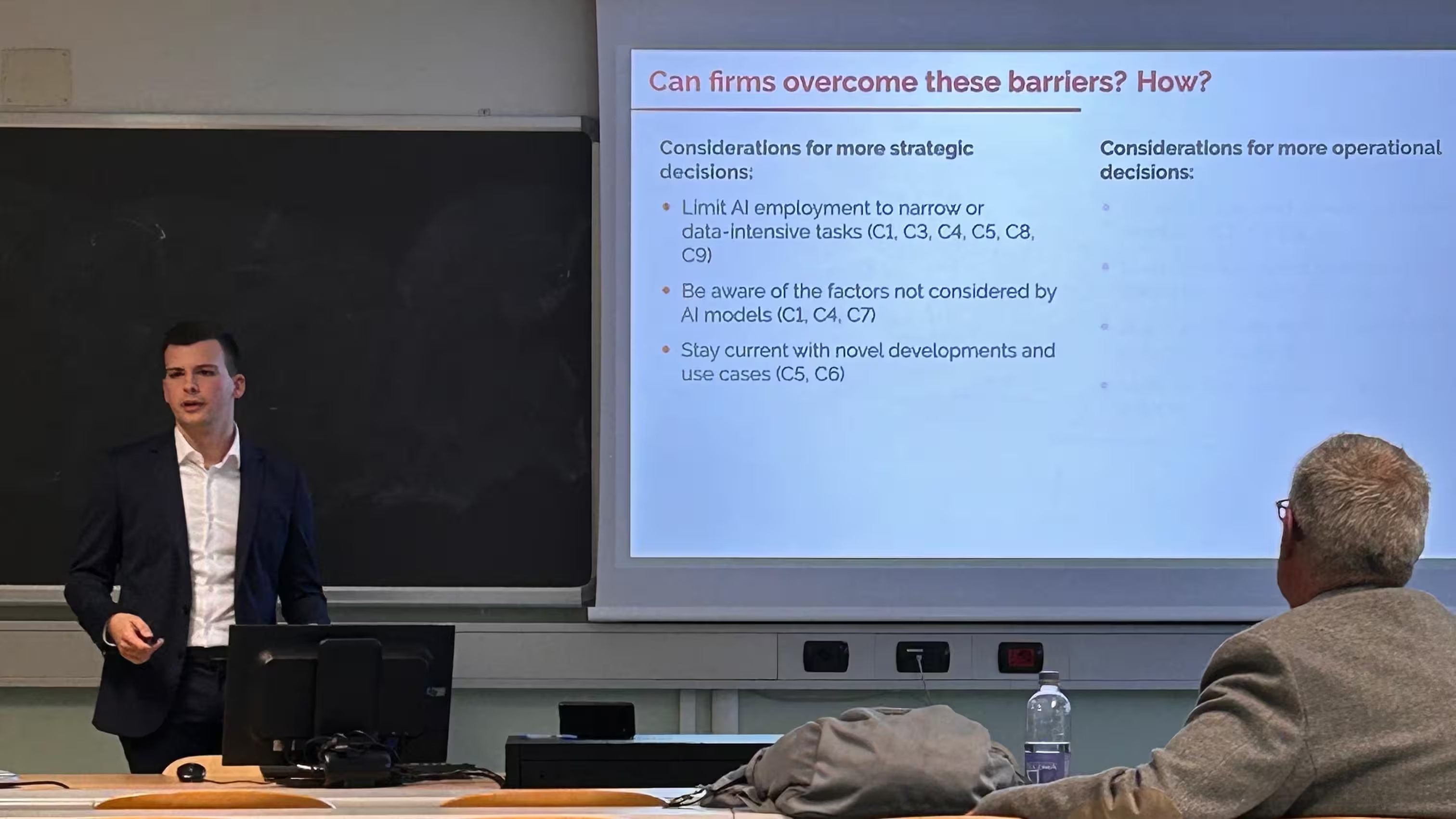
This day, I became the first Double Degree Master’s student of the faculty to graduate within two years. I will always remember my parents’ reactions when the commission assigned me the Laude.
Wrap-up
I was quite surprised to discover that, despite the AI hype, companies are not currently using AI applications to make strategic decisions. Many mentioned limited cognitive capabilities and reluctance about AI automation as the main elements hindering strategic adoption.
Instead, many companies are satisfied to apply Machine Learning (Deep Learning in particular) applications for decisions that:
- happen routinely
- are based solely on data
- can be structured and automated
- improve their accuracy through examples
- wouldn’t cause much harm if they were mistaken
Let’s see if this will change in the next years!
Bonus: sharing the thesis and slideshow templates
After celebrating with friends and family, I still had something to do: giving back to the LaTeX community. For my thesis and slideshow, I took inspiration from the high-quality LaTeX theses shared over the years.
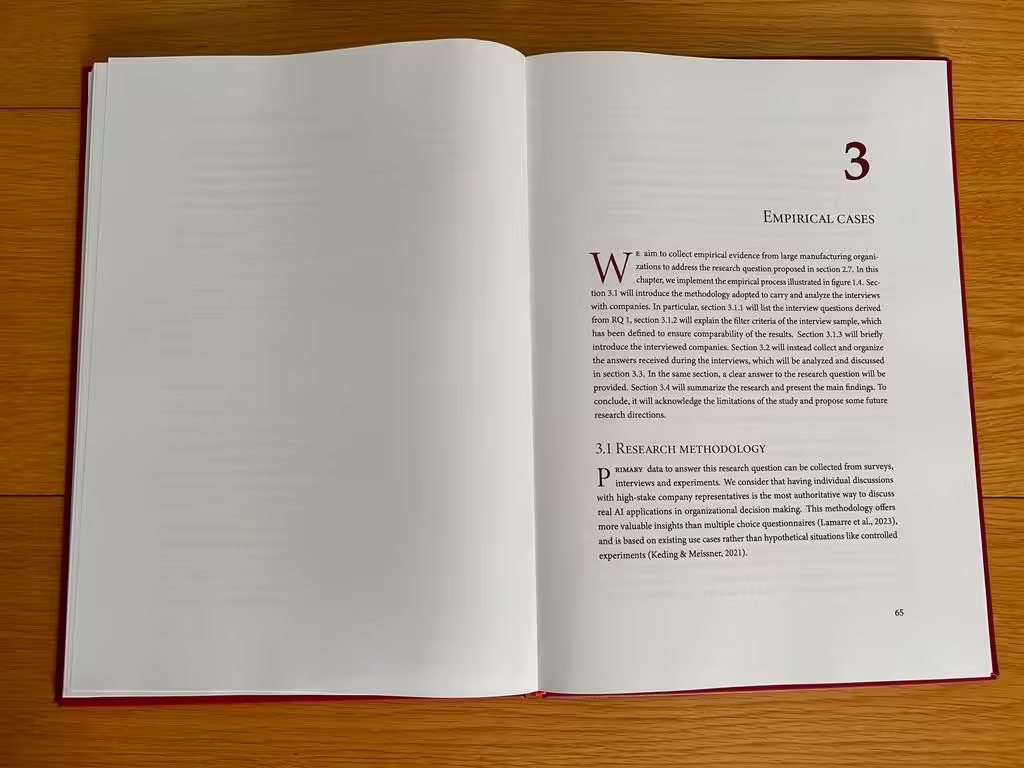
If you would like to do the same, the template is freely available on GitHub.
Read the full text
If you are interested in reading the full text, you can find it in the University of Padua website. Below you can find the BibTeX citation entry, just in case:
@mastersthesis{COLETTO2023,
author = {Coletto, Giacomo},
title = {The Impact of Artificial Intelligence on Strategic and Operational Decision Making},
school = {Università degli Studi di Padova},
url = {https://hdl.handle.net/20.500.12608/55202},
year = {2023}
}